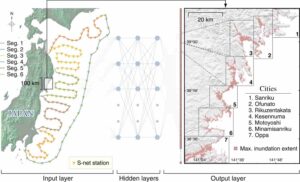
Detailed predictions about how an approaching tsunami will impact the northeastern coastline in Japan can be made in fractions of a second rather than half an hour or so—buying precious time for people to take appropriate action. This potentially life-saving technology exploits the power of machine learning.
The catastrophic tsunami that struck northeast Japan on March 11, 2011 claimed the lives of about 18,500 people. Many lives might have been saved if early warning of the impending tsunami had included accurate predictions of how high the water would reach at different points along the coastline and further inland.
The coast now boasts the world’s largest network of sensors for monitoring movement of the ocean floor. The 150 offshore stations making up this network provide early warning of tsunamis. But to be meaningful, the data generated by the sensors needs to be converted into tsunami heights and extents along the coastline.
This usually requires numerically solving difficult nonlinear equations, which typically takes about 30 minutes on a standard computer. But the 2011 tsunami hit some parts of the coast a mere 45 minutes after the earthquake.
Now, Iyan Mulia of the RIKEN Prediction Science Laboratory and co-workers have used machine learning to cut the calculation time to less than one second.
“The main advantage of our method is the speed of predictions, which is crucial for early warning,” explains Mulia. “Conventional tsunami modeling provides predictions after 30 minutes, which is too late. But our model can make predictions within seconds.”
Since tsunamis are rare occurrences, the team trained their machine-learning system using more than 3,000 computer-generated tsunami events. They then tested it with 480 other tsunami scenarios and three actual tsunamis. Their machine-learning-based model could achieve comparable accuracy at only 1% the computational effort.
The same deep-learning approach could be used for other disaster scenarios where time is of the essence. “The sky’s the limit—you can apply this method to any kind of disaster predictions where the time constraint is very limited,” says Mulia, who first became interested in studying tsunamis after the 2004 Indian Ocean tsunami devastated coastal regions in his home country of Indonesia. “I’m now working on a storm surge prediction, also using machine learning.”
The work is published in the journal Nature Communications.
Mulia notes that the method is only accurate for large tsunamis that are higher than about 1.5 meters, so he and his team are now seeking to improve its accuracy for smaller tsunamis.
Reference:
Iyan E. Mulia et al, Machine learning-based tsunami inundation prediction derived from offshore observations, Nature Communications (2022). DOI: 10.1038/s41467-022-33253-5
Note: The above post is reprinted from materials provided by RIKEN.